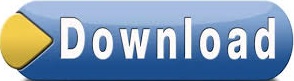

GEOMETRY THE SAME LINE M1 AND M2 PRO
This means when two lines are perpendicular to each other, the product of their slopes is -1, i.e., if m is the slope of L 1, then the slope L 2 ⏊ to it is (-1/m). So expect the M1 Pro to still come in around 40 percent faster than the M2. Let’s say this point M divides PQ in the ratio of n:1. Let M be the point on the line segment joining P and Q. Important: when determining the gradient of a line using the coefficient of x, make sure the given equation is. Let points P (x 1, y 1 ) and Q (x 2, y 2 ) be any two points on the curve represented by ax + by + c = 0. Let ax + by + c = 0 be a first-degree equation in x,y where a, b, and c are constant.
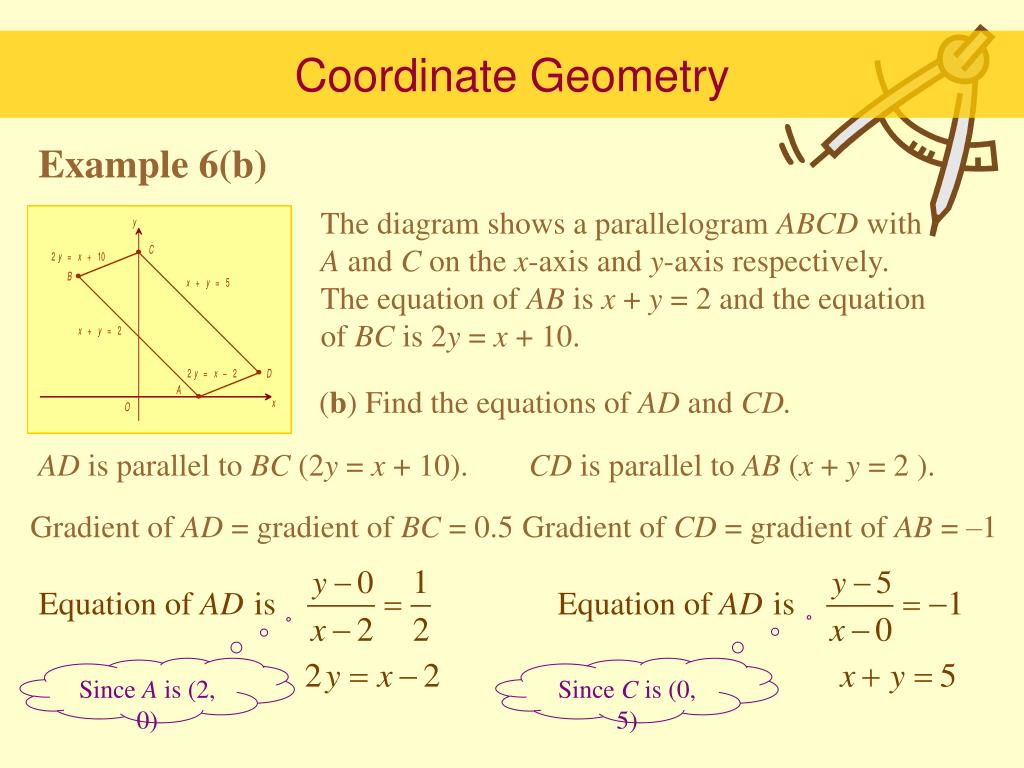
Let’s throw light on the coordinate geometry to prove that every first-degree equation in x, y represents a straight line. We define a straight line as a curve where every point on the line segment joining any two points lies on it. Oblique or slanting lines: The lines drawn in a slanting position are termed as oblique or slanting lines. Vertical lines: The lines drawn vertically Horizontal lines: The lines drawn horizontally A straight line can be further differentiated into horizontal, vertical, or slanted. This postulate, together with the definition of parallel lines, requires that one of three possibilities exist for any two coplanar lines, and : 1.and have no points in common. A straight line is devoid of curves and does not have any curve on it. It can also be said to be a combination of endless points joined on both sides of a point. The straight line extends to both sides.Ī straight line can be defined as an endless one-dimensional figure with no width.
GEOMETRY THE SAME LINE M1 AND M2 FULL
Now if we add those sides then we would be looking at full geometric relations where adding up lines will make up different shapes, that is a scientific notion but Mathematically any fixed line or curve on the line segment can be defined as a straight line.Ī line with zero or no curves or a structure with infinite length devoid of any curves is defined as a straight line. Now several examples of straight lines would be railway tracks, ruler (to measure), even parts of a mirror can be called a straight line. The later one takes significantly less computational time and converges much faster when compared to others.We come across many things daily which are completely straight and aligned along the similar lines or in parallel setting with the other line of the same length or different measurements but straight line. or we can use linear algebra techniques to find optimal theta. We can use iterative algorithms like gradient descent, Newton’s method etc.

Minimizing cost function: Now that we know our cost function, our next goal is to minimize it. It basically means that there shouldn’t be any relation between residuals w.r.t time.=> No autocorrelation. In the above derivation, we assumed that residuals( ε) have a normal distribution and hence normality of residuals.Īlso, as we assumed that ε(i)’s are independent and identically related((i.i.d), Residuals should also be independent and identically distributed. There can be other approaches that might infer the same end result.Īssumptions we made to choose OLS as our cost function: Note: This is one approach that justifies choosing OLS as a cost function. This expression is nothing but our OLS(Ordinary Least Squares) cost function.
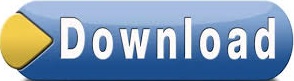